1.code
Reference library file
from __future__ import absolute_import, division, print_function, unicode_literals
import numpy as np
import pandas as pd
import tensorflow as tf
from tensorflow import feature_column
from tensorflow.keras import layers
from sklearn.model_selection import train_test_split
Load data set and generate data frame resource handle
# Download and load the heart.csv dataset into a data frame
path_data = "E:/pre_data/heart.csv"
dataframe = pd.read_csv(path_data)
The data format of panda dataframe is transformed into the data set format of TF. Data
# Copy the data frame, id(dataframe)! =id(dataframe_new)
dataframe_new = dataframe.copy()
# Get the target property from the dataframe_new data
labels = dataframe_new.pop('target')
# To build the data in the Dataset memory
dataset = tf.data.Dataset.from_tensor_slices((dict(dataframe_new), labels))
# The degree of confusion that will break up the data
dataset = dataset.shuffle(buffer_size=len(dataframe_new))
# Number of datasets to remove from the dataset
dstaset = dataset.batch(100)
# Specify the number of times the dataset should be duplicated
dataset = dataset.repeat(2)
2.suffle, batch and repeat
2.1 shuffle method/function
2.1.1 implementation process of shuffle function
Shuffle is a function used to scramble the data set, that is, shuffle the data. This method is very useful in training data
dataset = dataset.shuffle(buffer_size)
Parameter buffer_ The larger the size value is, the more chaotic the data is. The specific principle is as follows
Suppose buffer_ Size = 9, that is to say, first take out 9 data from the dataset and “drag” them to the buffer area, and each sample of subsequent training data will be obtained from the buffer area
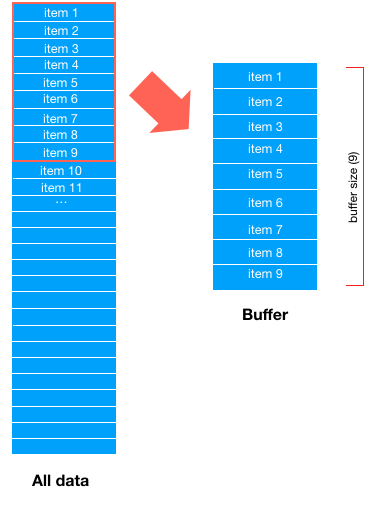
For example, take a data item 7 from the buffer area. Now there are only 8 data in the buffer area
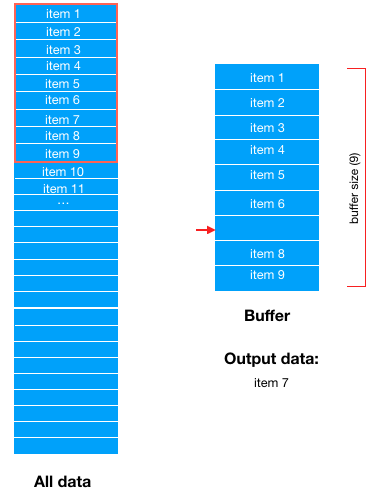
Then take out a piece of data (item10) from the dataset in order and “drag” it to the buffer area again to fill the gap
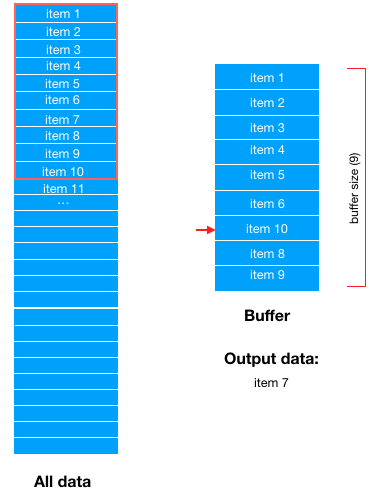
Then, when training data, one data is randomly selected from the buffer area; The buffer area forms a data vacancy
It should be noted that a data item here is just an abstract description, which is actually a Bach_ The size of the data
In fact, we can find that buffer actually defines the size of a data pool, buffer size. When the data is taken from the buffer, samples will be extracted from the source data set to fill the gaps in the buffer
2.1.2 parameters of shuffle method
buffer_ The size = 1 dataset will not be scrambled
buffer_ Size = the number of samples in the data set, randomly scrambling the whole data set
buffer_ size > The number of samples in the data set is randomly scrambled
Shuffle is an important means to prevent data from over fitting. However, improper buffer size will lead to meaningless shuffle. Please refer to the importance of buffer for details_ size in shuffle()
2.2 repeat method/function
The repeat method restarts the dataset when the data after the group is read. To limit the number of epochs, you can set the count
parameter
In order to match the output times, the default repeat() is empty
The repeat function is similar to epoch
The current optimizer SGD is the abbreviation of stochastic gradient descent, but it does not mean that it is based on a sample or mini batch
What does batch epoch iteration stand for
(1) Batch size: batch size. In deep learning, SGD training is generally used, that is, each training takes batch size samples in the training set
(2) Iteration: one iteration is equal to one training with batchsize samples
(3) Epoch: one epoch is equal to using all the samples in the training set to train once. Generally speaking, the value of epoch is the number of rounds of the whole data set
For example, if the training set has 500 samples and batchsize = 10, then the training set has a complete sample set: iteration = 50, epoch = 1.
– – –
Author: bboysky45
source: CSDN
original text: https://blog.csdn.net/qq_ 18668137/article/details/80883350
copyright notice: This is the original article of the blogger, please attach the blog link if you want to reprint it
2.3 batch method/function
The batch size of the data fed into the neural network at one time
3. The relationship among shuffle, repeat and batch
On the official website, it is explained that the use of repeat before shuffle can effectively improve the performance, but it blurs the epoch of data samples
In fact, you can understand that shuffle has reset the source dataset before fetching
That is, repeat before shuffle. TF will multiply the data set by the number of repeats, and then scramble it as a data set
dataset = dataset.shuffle(buffer_size=10000)
dataset = dataset.batch(32)
dataset = dataset.repeat(num_epochs)